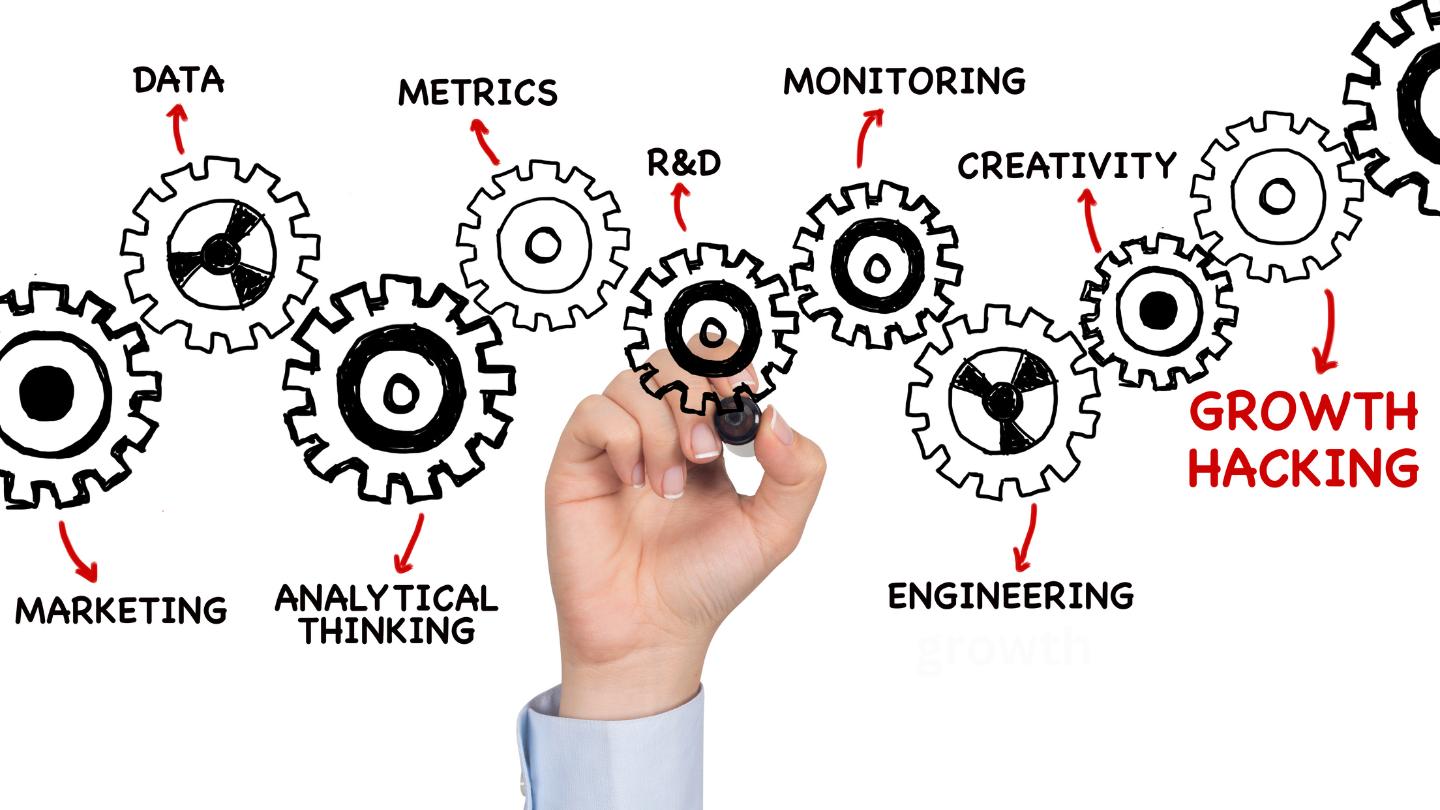
Adopting AI and Data Analytics as Growth Engineering Factors
Despite substantial investments in research-driven marketing and scalable product development, over 70% of businesses struggle to achieve sustainable sales and revenue growth, primarily due to a lack of actionable insights into what truly works.
Many organizations fail to validate their strategies through measurable testing, neglect ROI analysis, and fall into the trap of intuition-driven decisions or outdated models, leading to misaligned efforts and missed opportunities.
The path forward lies in leveraging AI methodology, purpose-based ML models, and data analytics to drive strategic growth. These technologies empower companies with measurable innovation, testing of research and MVPs, intelligent experimentation, and real-time optimization, enabling them to adapt faster, scale smarter, and thrive in dynamic markets.
If you wish to turn uncertainty into precision and potential into performance, learn from us to know how AI and data analytics are transforming growth engineering.
How Does Growth Engineering Work by Leveraging AI?
You’ve launched a promising mobile app. The UI is slick, the reviews are positive, and you hit 1,000 downloads within the first month. But then growth stalls. Nothing moves the needle, no matter what you try, running a few ads, tweaking the homepage, or posting on social media. You're not alone. This is where most apps flatline.
Instead of guessing what to do next, you apply growth engineering powered by AI and data analytics to kick-start the download. Growth engineering uses real-time data and testing to determine what works and what does not.
Let us explain it with a step-by-step example.
Step 1: Diagnose with Data
You feed your user behavior data into a machine learning model, revealing a key insight: 40% of users drop off within the first 60 seconds. Heatmaps show they’re stuck trying to figure out one specific onboarding screen.
Step 2: Intelligent Experimentation
Using A/B testing, you launch three new onboarding variations, each guided by user flow predictions. You don’t guess—you let the data decide.
Step 3: Real-Time Optimization
You run predictive analytics using an AI/ML model, suggesting the ideal time to prompt for a premium upgrade. After the third successful use, you implement the change. Conversion rate jumps by 27%.
Step 4: Scaling What Works
Your AI tool clusters users by region, time zone, and behavior, discovering that South Asian users are three times more likely to convert when the app is marketed as a productivity tool, not just a tracker.
Based on these findings, you tailor the ad messaging and landing pages, and growth picks up again, this time sustainably.
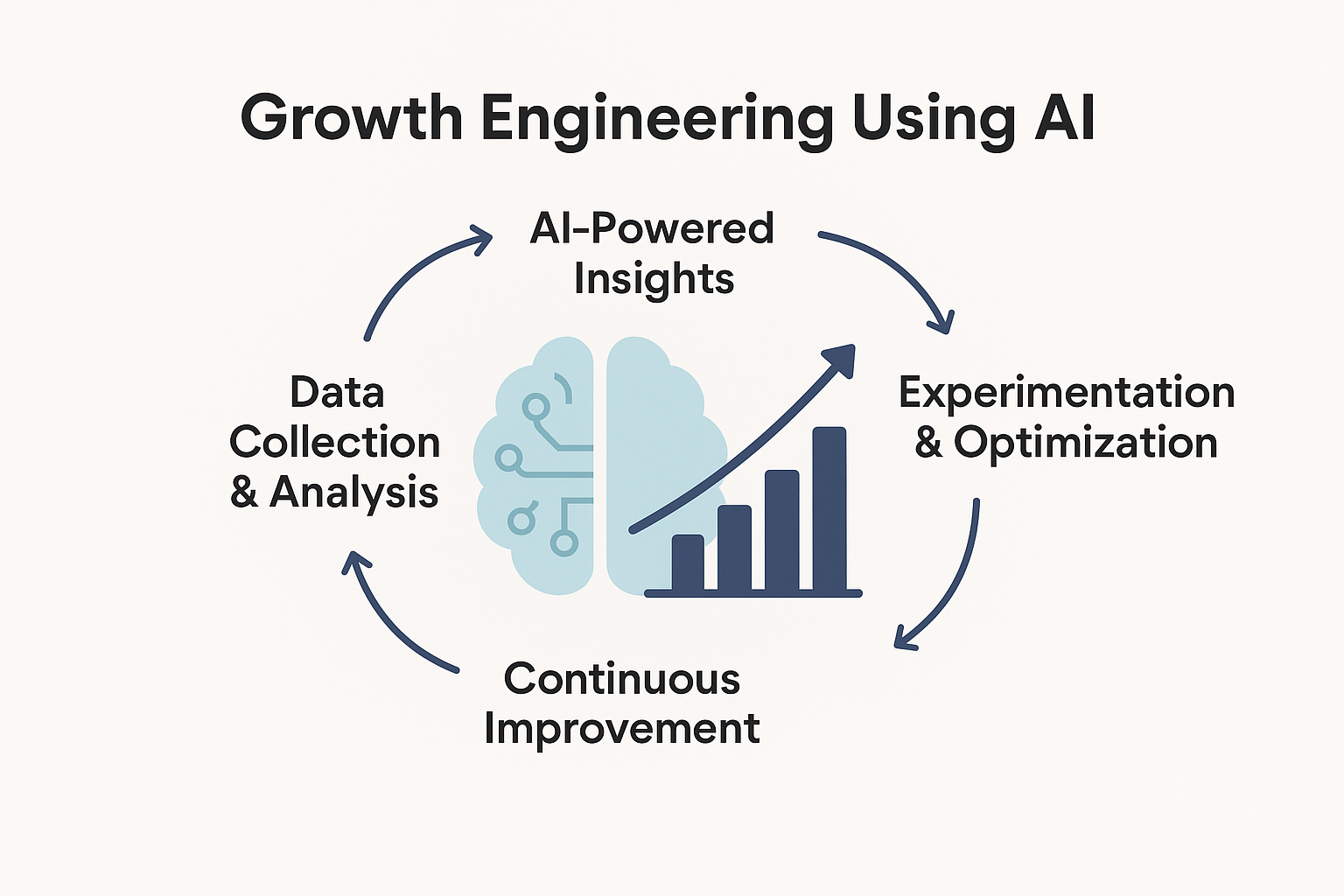
The Role of AI in Growth Engineering
AI is the main engine behind automation, prediction, and personalization capabilities that supercharge growth engineering strategies.
It replaces manual guesswork with smart systems that optimize every step of the customer journey.
Growth engineering is only effective when you can prove its impact. That’s where metrics come in.
But tracking random KPIs is not enough. You need to connect data and AI efforts directly to outcomes like revenue, retention, and user satisfaction.
Data Analytics- The Backbone of AI
In growth engineering, data analytics plays two crucial roles. First, it decodes real-time events, such as user behavior, feature performance, and campaign ROI.
Second, it uncovers patterns that allow companies to predict what will likely happen next and prepare accordingly.
While AI predicts and automates, data analytics validates and explains. Analytics is how you interpret the past to inform the future.
Behind every growth-focused company today is a stack of tools that help teams collect, process, analyze, and act on data. Failing to manage them can quickly lead to information silos, duplicated effort, and inconsistent decisions.
Data Analytics Tools
The growth stack begins by capturing user interactions across websites and apps. This raw data is sent to cloud warehouses like Snowflake or BigQuery for fast processing. Tools like DBT or Airbyte then clean and organize the data.
Analytics platforms turn it into easy-to-read insights, and finally, AI and automation use this data to manage things like product suggestions, retention emails, or smart pricing in real time.
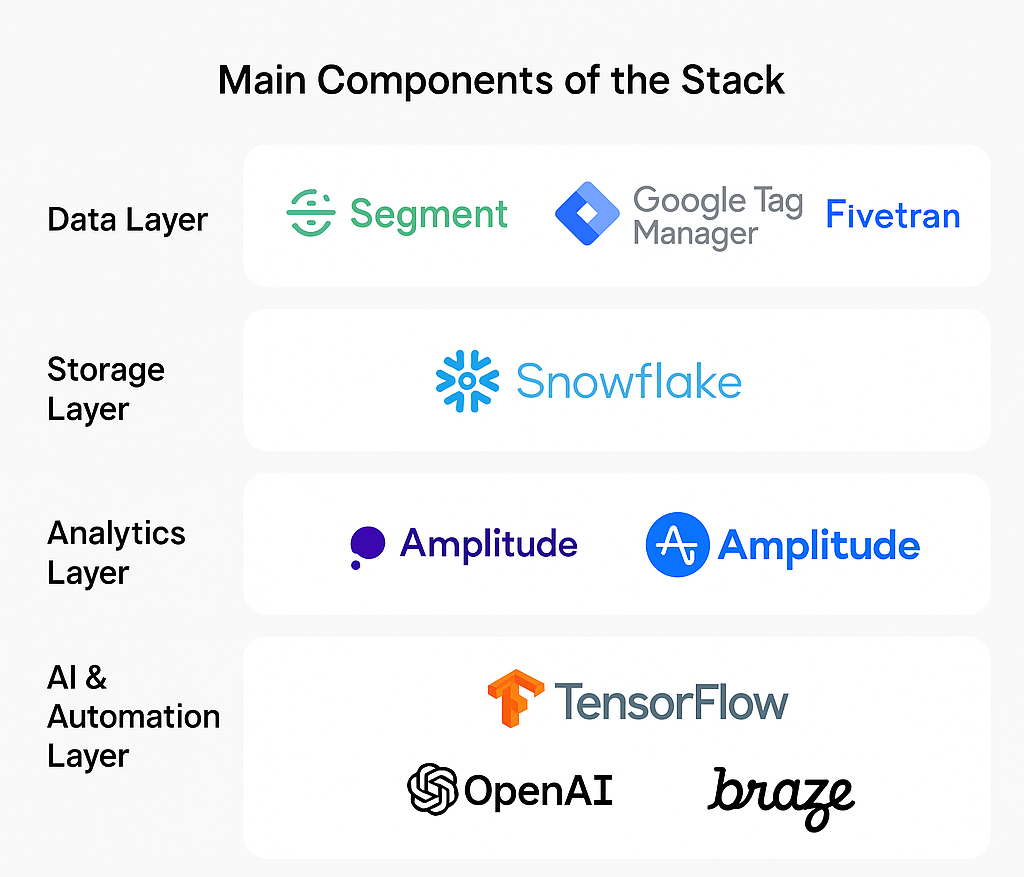
How to Apply Growth Engineering to Your Operations
Here are the core pillars of Growth Engineering, widely recognized in modern product and business growth strategies, especially when powered by AI, data, and experimentation
1. Data-Driven Mindset
Growth engineers do not make decisions based on gut feeling. They rely on metrics, conversion rates, churn rates, customer lifetime value (CLV), acquisition cost, engagement levels, and more.
For example, if users drop off after signing up, a growth engineer will use tools like Google Analytics or Mixpanel to find out where and why.
Collecting and analyzing user data to uncover hidden patterns and friction points may help bring an edge.
2. A/B Testing and Experimentation
Engineers test multiple versions of a feature, email, or design (A/B tests) to find what performs best.
For example, Netflix might test 10 versions of a homepage banner to see which ones get more clicks. The one that wins becomes the new standard.
Testing new landing page CTAs is essential to validate assumptions and optimize key metrics.
3. Full-Funnel Focus
Growth engineering is not just about marketing or product. It targets the entire growth funnel.
- Acquisition: How users find you.
- Activation: Their first experience.
- Retention: Why they stay.
- Revenue: How you earn.
- Referral: How they bring others.
It is a continuous cycle of acquiring new customers and retaining previous ones to power the revenue and growth cycle.
4. AI-Powered Personalization & Optimization
Using ML and AI to personalize experiences, predict churn, optimize timing, and refine real-time recommendations.
Growth engineers have the technical capabilities to build and ship features, automate workflows, and set up tracking systems. They work with:
- APIs for automating marketing.
- SQL or Python for analyzing user behavior.
- Firebase or Amplitude for tracking app events.
- Feature flags to roll out experiments safely.
Dynamic pricing or push notification timing using AI may help engage customers hooked to your landing page, decreasing the risk of abandonment.
5. Scalable Systems & Automation
One of the key factors of growth engineering is adopting scalable technology and systems. Building repeatable, automated systems for experimentation, user acquisition, retention, and monetization will help proliferate the customer acquisition and retention cycle.
You can use AI and ML-based systems and tools to automate the onboarding experiments pipeline to ensure customer inflow. Similarly, continuously iterating the product based on user feedback and behavior will improve retention and customer satisfaction.
How to Curb the Challenges along the Growth Engineering Route
Despite the potential, adopting AI and analytics is not plug-and-play. The biggest roadblocks are not always technical. They are cultural and operational.
The biggest challenges with business process automation are messy data, resistance to change, and a lack of the right talent. Yet, overcoming these hurdles is what separates high-growth companies from lifeless ones.
What Does the Future of Growth Engineering Hold?
AI-driven growth systems will run thousands of micro-experiments without human input, enabling systems to optimize messaging, pricing, and user flow dynamically based on continuous feedback loops.
Another rising trend is the use of IoT data to personalize experiences in physical environments.
Similarly, ethical AI and data privacy are becoming non-negotiable. Consumers want personalization without feeling spied on, so companies must be transparent and compliant.
Lastly, the no-code AI revolution is democratizing industrial growth. Marketers, product managers, and even customer service teams can build and deploy required machine learning models without writing code. However, be wary of making seemingly AI mistakes that could kill your business.
If your company is not using AI, you are not just falling behind, but handing market share to those who do. Ally with Iowa software teams using offshore full-stack devs continuously working to upgrade business with AI and data analytics for the best customer experience.
Related Post
RECOMMENDED POSTS
RECOMMENDED TOPICS
TAGS
- ai
- artificial intelligence
- agentic ai
- deepseek
- machine learning
- llm
- ai development
- openai
- gpt
- ai/ml
- data science
- chatgpt
- open source
- modern medicine
- growth engineering
- growth hacks
- data roles
- data analyst
- data scientist
- data engineer
- data visualization
- productivity
- database optimize
- database management
- sql query
- data isolation
- db expert
- healthcare
- automation
- bpa
- artificial intelligene
- qwenlm
- xai
- ai model
- dynamic data
- static data
- empathy
- it development
- leadership
- llm models
- climate change
- test
ABOUT
Stay ahead in the world of technology with Iowa4Tech.com! Explore the latest trends in AI, software development, cybersecurity, and emerging tech, along with expert insights and industry updates.
Comments(0)
Leave a Reply
Your email address will not be published. Required fields are marked *